----Amey Acharya, Msc Statistics & Data Science
Recently when our first semester started, we were in search of the topics for our research treatise projects. One day our distribution theory teacher introduced us to a new concept "MIXTURE DISTRIBUTIONS".
Now this was something interesting! We have tossed so many coins in our life and applied binomial theorem that how many times we can get heads and tails. Whenever my mother asked me to buy a check shirt, and the shopkeeper tried selling the non-check shirts I quickly remember the friendship of Geometric-Binomial-Negative Binomial distributions' friendship. (Geometric distribution describes the number of trials required to get first success. But this kid "Geometric" doesn't know when it comes to counting the trials to get multiple successes. Then "Negative Binomial" comes into action and it counts the number of trials required to get particular number of successes. And when we have to find vice versa i.e. when we have to find a number of successes in particular number of trials "Binomial" takes the responsibility.)
I knew about the friendship of these 3 distributions. But I was amazed that in Statistics, this friendship does not limit to these 3 distributions only. But we come to know about the "Yarana" of these distributions while studying mixture and compound distributions.
MIXTURE DISTRIBUTIONS:
The Ganpati Visarjan at Juhu Beach:
Imagine Ganpati Visarjan scene at Juhu Beach. Two major groups "Andheri cha Raja" and "Vile-Parle cha Raja" have arrived at the beach for Visarjan. Each group will have its unique style of celebrating the moment.
"Andheri cha Raja" and "Vile-Parle Cha Raja” both arriving with their own processions, each representing a different distribution in terms of their music style, energy, and the pace of their processions.
For a moment, Andheri cha Raja group might have a fast, more upbeat Dhol-Tasha style, while Vile Parle Cha Raja group might bring a slower, more rhythmic beat. Each group has its own style, just as different probability distributions have their own characteristics (mean, variance, spread, etc.).
But not every group will have the same type of rush or chaos. In fact, Andheri cha raja will have massive crowd and will contribute about 70% of total chaos on the beach whereas Vile-Parle cha Raja will contribute only 30%. These percentages are weights (in theory we called it as mixing probabilities) of distributions which represent how much a group(variable) contributes to overall experience(distribution).
The experience you get is a combination or mixture, of the two distribution based on characteristics of two processions. Music of Andheri cha Raja has the larger share in this mixed “distribution” due to its larger crowd, but Vile Parle Cha Raja’s unique style still adds its own flavor, and we see the "friendship" between these two distributions working on the scene of Ganpati Visarjan.
In statistical language:
Each group is a component distribution to the derived mixture distribution due to its own style and characteristics.
The weights (70% for Andheri cha Raja and 30% for Vile Parle Cha Raja) represent the influence of each procession to the overall experience.
The final mixture distribution is the overall joy that you experience at Juhu Beach, combining the sound, energy and rhythm of both processions according to their weights.
So, just like in mixture distributions, where multiple distributions contribute to derive a new distribution, here both processions contribute to the enjoyable atmosphere at Juhu Beach, with each bringing its own crowd and devotion to the Ganpati Visarjan celebration!
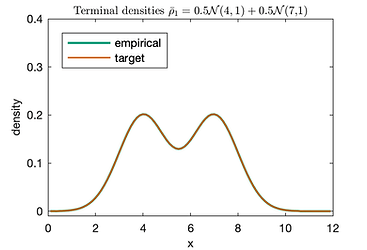
Compound Distributions:
Tossing a coin and rolling a die are the classic, most used and simple examples used in statistics to explain the general probability concepts. So, Let’s understand compound distribution with a die and a coin with the help of a story (as I am a storyteller also).
As a storyteller I want to take you to a funfair which is held in the basement of the college near our canteen. Imagine you’re at a game stall during a fair, and you’ve come across this interesting game. The rules are simple but layered, just like the compound distribution concept!
Step 1: Roll the Die
The number of the heads you'll get after tossing the coins will decide which gift you'll win. But here’s the twist: The game starts with rolling a die. The number you get on the die decides how many coins you’ll toss afterwards. If you roll a 4, you’ll toss 4 coins; if you roll a 2, only 2 coins. So, your coin-tossing depends on what number comes up on the face of your die.
Step 2: Toss the Coins
Now, with the number of coins decided, you toss them and count how many heads came up. But this count of heads depends entirely on how many coins you've tossed based on your die roll. The more coins you have, the higher your chance of getting heads, just as each trial in the die influences the overall game outcome.
So, we have two steps here: first, rolling the die to determine the number of coins, and second, counting heads from those coin tosses. In statistical language, this two-layered process is a compound distribution.
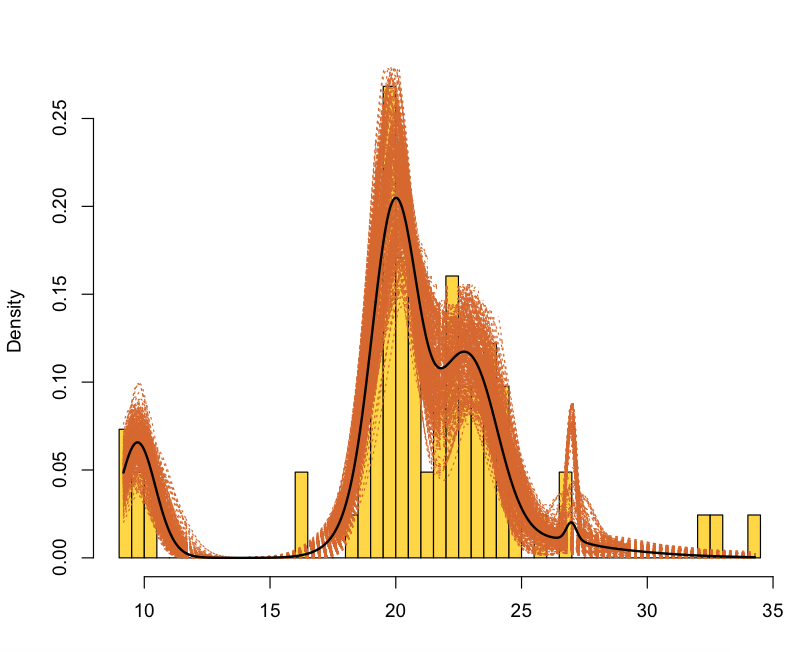
In Statistical Terms:
First Layer (Die Roll): This is like a “distribution within a distribution.” The die roll is our first layer. It’s the outer distribution that determines the number of coins.
Second Layer (Coin Tosses): Once the number of coins is fixed, we can think of the total heads as the inner distribution. Each possible die outcome (like rolling a 4 and tossing 4 coins) creates a new inner distribution for heads.
The Compound Effect
So, the overall distribution of heads is a "compound distribution" which is a blend created by one distribution (the die) stepping into another (the coins).
In other words, the compound distribution here reflects a situation where one random variable (the die roll) determines the behavior of another (the coin tosses), creating an overall distribution that captures this layered randomness.
In a compound distribution, a parameter of one probability distribution is treated as a random variable that follows another probability distribution.
I hope you’ve found this blog interesting and informative. Getting information in an interesting is itself interesting. Now as a reader many of you will feel that I shall not be a normal person. Well, I don’t know about that because I haven’t done my Shapiro test yet.
Thank You!!!
Great explanation!!!!
Excellent way to explain concepts
Nice concept explanation
Keep posting informative blogs